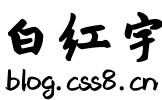
本文共 8514 字,大约阅读时间需要 28 分钟。
呆鸟云:“翻译不易,要么是一个词反复思索,要么是上万字一遍遍校稿修改,只为给大家翻译更准确、阅读更舒适的感受,呆鸟也不求啥,就是希望各位看官如果觉得本文有用,能给点个在看或分享给有需要的朋友,这就是对呆鸟辛苦翻译的最大鼓励。”
描述性统计
Series 与 DataFrame 支持大量计算描述性统计的方法与操作。这些方法大部分都是 sum()
、mean()
、quantile()
等聚合函数,其输出结果比原始数据集小;此外,还有输出结果与原始数据集同样大小的 cumsum()
、 cumprod()
等函数。这些方法都基本上都接受 axis
参数,如, ndarray.{sum,std,…}
,但这里的 axis
可以用名称或整数指定:
Series:无需
axis
参数DataFrame:
"index",即
axis=0
,默认值"columns", 即
axis=1
示例如下:
In [77]: dfOut[77]: one two threea 1.394981 1.772517 NaNb 0.343054 1.912123 -0.050390c 0.695246 1.478369 1.227435d NaN 0.279344 -0.613172In [78]: df.mean(0)Out[78]:one 0.811094two 1.360588three 0.187958dtype: float64In [79]: df.mean(1)Out[79]:a 1.583749b 0.734929c 1.133683d -0.166914dtype: float64
这些方法都支持 skipna
,这个关键字指定是否要把缺失数据排除在外,默认值为 True
。
In [80]: df.sum(0, skipna=False)Out[80]:one NaNtwo 5.442353three NaNdtype: float64In [81]: df.sum(axis=1, skipna=True)Out[81]:a 3.167498b 2.204786c 3.401050d -0.333828dtype: float64
结合广播机制或算数操作,可以描述不同统计过程,比如标准化,即渲染数据零均值与标准差 1,这种操作非常简单:
In [82]: ts_stand = (df - df.mean()) / df.std()In [83]: ts_stand.std()Out[83]:one 1.0two 1.0three 1.0dtype: float64In [84]: xs_stand = df.sub(df.mean(1), axis=0).div(df.std(1), axis=0)In [85]: xs_stand.std(1)Out[85]:a 1.0b 1.0c 1.0d 1.0dtype: float64
注 : cumsum()
与 cumprod()
等方法保留 NaN
值的位置。这与 expanding()
和 rolling()
略显不同,详情请参阅本文。
In [86]: df.cumsum()Out[86]: one two threea 1.394981 1.772517 NaNb 1.738035 3.684640 -0.050390c 2.433281 5.163008 1.177045d NaN 5.442353 0.563873
下面是常用函数汇总表。每个函数都支持 level
参数,仅在数据对象为结构化 Index 时使用。
函数 | 描述 |
---|---|
count | 统计非空值数量 |
sum | 汇总值 |
mean | 平均值 |
mad | 平均绝对偏差 |
median | 算数中位数 |
min | 最小值 |
max | 最大值 |
mode | 众数 |
abs | 绝对值 |
prod | 乘积 |
std | 贝塞尔校正的样本标准偏差 |
var | 无偏方差 |
sem | 平均值的标准误差 |
skew | 样本偏度 (第三阶) |
kurt | 样本峰度 (第四阶) |
quantile | 样本分位数 (不同 % 的值) |
cumsum | 累加 |
cumprod | 累乘 |
cummax | 累积最大值 |
cummin | 累积最小值 |
注意:Numpy 的 mean
、std
、sum
等方法默认不统计 Series 里的空值。
In [87]: np.mean(df['one'])Out[87]: 0.8110935116651192In [88]: np.mean(df['one'].to_numpy())Out[88]: nan
Series.nunique()
返回 Series 里所有非空值的唯一值。
In [89]: series = pd.Series(np.random.randn(500))In [90]: series[20:500] = np.nanIn [91]: series[10:20] = 5In [92]: series.nunique()Out[92]: 11
数据总结:describe
describe()
函数计算 Series 与 DataFrame 数据列的各种数据统计量,注意,这里排除了空值。
In [93]: series = pd.Series(np.random.randn(1000))In [94]: series[::2] = np.nanIn [95]: series.describe()Out[95]:count 500.000000mean -0.021292std 1.015906min -2.68376325% -0.69907050% -0.06971875% 0.714483max 3.160915dtype: float64In [96]: frame = pd.DataFrame(np.random.randn(1000, 5), ....: columns=['a', 'b', 'c', 'd', 'e']) ....:In [97]: frame.iloc[::2] = np.nanIn [98]: frame.describe()Out[98]: a b c d ecount 500.000000 500.000000 500.000000 500.000000 500.000000mean 0.033387 0.030045 -0.043719 -0.051686 0.005979std 1.017152 0.978743 1.025270 1.015988 1.006695min -3.000951 -2.637901 -3.303099 -3.159200 -3.18882125% -0.647623 -0.576449 -0.712369 -0.691338 -0.69111550% 0.047578 -0.021499 -0.023888 -0.032652 -0.02536375% 0.729907 0.775880 0.618896 0.670047 0.649748max 2.740139 2.752332 3.004229 2.728702 3.240991
此外,还可以指定输出结果包含的分位数:
In [99]: series.describe(percentiles=[.05, .25, .75, .95])Out[99]:count 500.000000mean -0.021292std 1.015906min -2.6837635% -1.64542325% -0.69907050% -0.06971875% 0.71448395% 1.711409max 3.160915dtype: float64
一般情况下,默认值包含中位数。
对于非数值型 Series 对象, describe()
返回值的总数、唯一值数量、出现次数最多的值及出现的次数。
In [100]: s = pd.Series(['a', 'a', 'b', 'b', 'a', 'a', np.nan, 'c', 'd', 'a'])In [101]: s.describe()Out[101]:count 9unique 4top afreq 5dtype: object
注意:对于混合型的 DataFrame 对象, describe()
只返回数值列的汇总统计量,如果没有数值列,则只显示类别型的列。
In [102]: frame = pd.DataFrame({'a': ['Yes', 'Yes', 'No', 'No'], 'b': range(4)})In [103]: frame.describe()Out[103]: bcount 4.000000mean 1.500000std 1.290994min 0.00000025% 0.75000050% 1.50000075% 2.250000max 3.000000
include/exclude
参数的值为列表,用该参数可以控制包含或排除的数据类型。这里还有一个特殊值,all
:
In [104]: frame.describe(include=['object'])Out[104]: acount 4unique 2top Yesfreq 2In [105]: frame.describe(include=['number'])Out[105]: bcount 4.000000mean 1.500000std 1.290994min 0.00000025% 0.75000050% 1.50000075% 2.250000max 3.000000In [106]: frame.describe(include='all')Out[106]: a bcount 4 4.000000unique 2 NaNtop Yes NaNfreq 2 NaNmean NaN 1.500000std NaN 1.290994min NaN 0.00000025% NaN 0.75000050% NaN 1.50000075% NaN 2.250000max NaN 3.000000
本功能依托于 select_dtypes
,要了解该参数接受哪些输入内容请参阅本文。
最大值与最小值对应的索引
Series 与 DataFrame 的 idxmax()
与 idxmin()
函数计算最大值与最小值对应的索引。
In [107]: s1 = pd.Series(np.random.randn(5))In [108]: s1Out[108]:0 1.1180761 -0.3520512 -1.2428833 -1.2771554 -0.641184dtype: float64In [109]: s1.idxmin(), s1.idxmax()Out[109]: (3, 0)In [110]: df1 = pd.DataFrame(np.random.randn(5, 3), columns=['A', 'B', 'C'])In [111]: df1Out[111]: A B C0 -0.327863 -0.946180 -0.1375701 -0.186235 -0.257213 -0.4865672 -0.507027 -0.871259 -0.1111103 2.000339 -2.430505 0.0897594 -0.321434 -0.033695 0.096271In [112]: df1.idxmin(axis=0)Out[112]:A 2B 3C 1dtype: int64In [113]: df1.idxmax(axis=1)Out[113]:0 C1 A2 C3 A4 Cdtype: object
多行或多列中存在多个最大值或最小值时,idxmax()
与 idxmin()
只返回匹配到的第一个值的 Index
:
In [114]: df3 = pd.DataFrame([2, 1, 1, 3, np.nan], columns=['A'], index=list('edcba'))In [115]: df3Out[115]: Ae 2.0d 1.0c 1.0b 3.0a NaNIn [116]: df3['A'].idxmin()Out[116]: 'd'
::: tip 注意
idxmin
与 idxmax
对应 Numpy 里的 argmin
与 argmax
。
:::
值计数(直方图)与众数
Series 的 value_counts()
方法及顶级函数计算一维数组中数据值的直方图,还可以用作常规数组的函数:
In [117]: data = np.random.randint(0, 7, size=50)In [118]: dataOut[118]:array([6, 6, 2, 3, 5, 3, 2, 5, 4, 5, 4, 3, 4, 5, 0, 2, 0, 4, 2, 0, 3, 2, 2, 5, 6, 5, 3, 4, 6, 4, 3, 5, 6, 4, 3, 6, 2, 6, 6, 2, 3, 4, 2, 1, 6, 2, 6, 1, 5, 4])In [119]: s = pd.Series(data)In [120]: s.value_counts()Out[120]:6 102 104 95 83 80 31 2dtype: int64In [121]: pd.value_counts(data)Out[121]:6 102 104 95 83 80 31 2dtype: int64
与上述操作类似,还可以统计 Series 或 DataFrame 的众数,即出现频率最高的值:
In [122]: s5 = pd.Series([1, 1, 3, 3, 3, 5, 5, 7, 7, 7])In [123]: s5.mode()Out[123]:0 31 7dtype: int64In [124]: df5 = pd.DataFrame({"A": np.random.randint(0, 7, size=50), .....: "B": np.random.randint(-10, 15, size=50)}) .....:In [125]: df5.mode()Out[125]: A B0 1.0 -91 NaN 102 NaN 13
离散化与分位数
cut()函数
(以值为依据实现分箱)及 qcut()函数
(以样本分位数为依据实现分箱)用于连续值的离散化:
In [126]: arr = np.random.randn(20)In [127]: factor = pd.cut(arr, 4)In [128]: factorOut[128]:[(-0.251, 0.464], (-0.968, -0.251], (0.464, 1.179], (-0.251, 0.464], (-0.968, -0.251], ..., (-0.251, 0.464], (-0.968, -0.251], (-0.968, -0.251], (-0.968, -0.251], (-0.968, -0.251]]Length: 20Categories (4, interval[float64]): [(-0.968, -0.251] < (-0.251, 0.464] < (0.464, 1.179] < (1.179, 1.893]]In [129]: factor = pd.cut(arr, [-5, -1, 0, 1, 5])In [130]: factorOut[130]:[(0, 1], (-1, 0], (0, 1], (0, 1], (-1, 0], ..., (-1, 0], (-1, 0], (-1, 0], (-1, 0], (-1, 0]]Length: 20Categories (4, interval[int64]): [(-5, -1] < (-1, 0] < (0, 1] < (1, 5]]
qcut()
计算样本分位数。比如,下列代码按等距分位数分割正态分布的数据:
In [131]: arr = np.random.randn(30)In [132]: factor = pd.qcut(arr, [0, .25, .5, .75, 1])In [133]: factorOut[133]:[(0.569, 1.184], (-2.278, -0.301], (-2.278, -0.301], (0.569, 1.184], (0.569, 1.184], ..., (-0.301, 0.569], (1.184, 2.346], (1.184, 2.346], (-0.301, 0.569], (-2.278, -0.301]]Length: 30Categories (4, interval[float64]): [(-2.278, -0.301] < (-0.301, 0.569] < (0.569, 1.184] < (1.184, 2.346]]In [134]: pd.value_counts(factor)Out[134]:(1.184, 2.346] 8(-2.278, -0.301] 8(0.569, 1.184] 7(-0.301, 0.569] 7dtype: int64
定义分箱时,还可以传递无穷值:
In [135]: arr = np.random.randn(20)In [136]: factor = pd.cut(arr, [-np.inf, 0, np.inf])In [137]: factorOut[137]:[(-inf, 0.0], (0.0, inf], (0.0, inf], (-inf, 0.0], (-inf, 0.0], ..., (-inf, 0.0], (-inf, 0.0], (-inf, 0.0], (0.0, inf], (0.0, inf]]Length: 20Categories (2, interval[float64]): [(-inf, 0.0] < (0.0, inf]]
发表评论
最新留言
关于作者
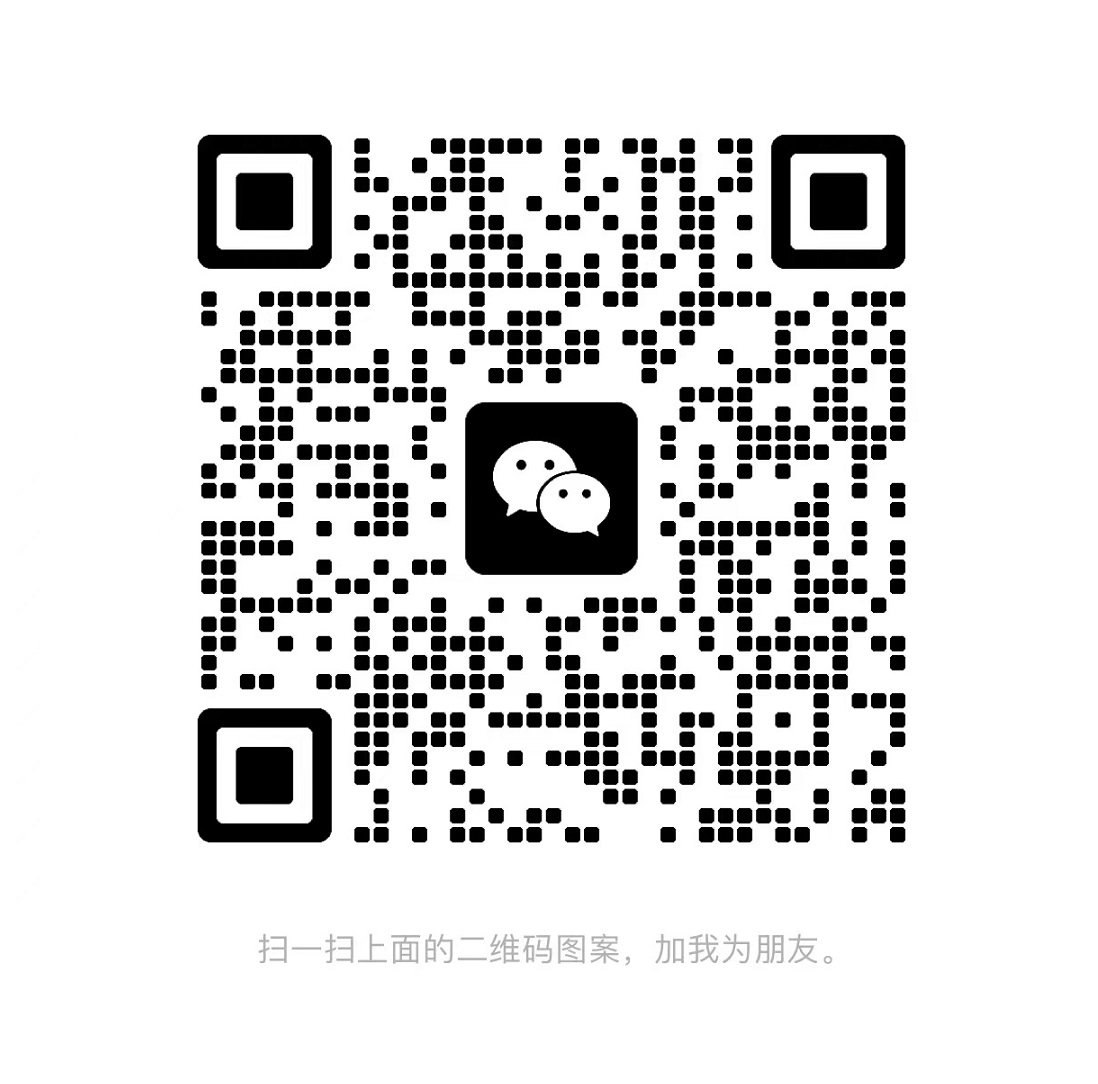